DONUT Objectives and Impact
“DONUT” is a project funded by the European Union. Its primary objective is to enhance the education and training of doctoral candidates, particularly in the fields of Brain-Computer Interface (BCI) and Electroencephalography (EEG) technologies. BCI detects brain signals, analyses them, and translates them into instructions that a computer can understand. BCIs vary in invasiveness, ranging from non-invasive methods such as EEG and Magnetic Resonance Imaging to partially invasive methods like Electrocorticography, and fully invasive methods like Microelectrode arrays. The project's mission is to establish a multidisciplinary and inter-sectoral Doctoral Network (DN) for talented researchers, specifically doctoral candidates (DCs), to equip them for research in EEG-based BCI applications. These BCI tools can serve various purposes, including communication, signal analysis, applications in healthcare (rehabilitation, neural prosthetics, diagnostics), industrial applications, and even in the entertainment sector (Virtual Reality, biometrics). Early scientific independence is one of key goals of PhD training programmes.
The crucial point of this research project is to enhance doctoral training at the European level and bolster Europe's innovation capacity through the establishment of a cutting-edge doctoral network within the European Research Collaboration on Neural Prostheses and Brain Research. Moreover, by tackling the challenges associated with modern human-machine interfaces, the project holds the potential to facilitate the co-development of innovative Brain-Computer Interface (BCI) technologies. It is the ambition of DONUT to build a strong and lasting network not only between the DCs but also between the participating beneficiaries and associated partners. DONUT researchers will benefit of a dense network of contacts with scientists acquired during network-wide training events, to improve their career prospects in the European and worldwide innovation sector, having the opportunity to become scientists employable in both the industrial and academic sectors. The participation of 7 industrial participants in research and training programmes will guarantee extensive inter-sectoral experience for the trainees and maximise the impact.
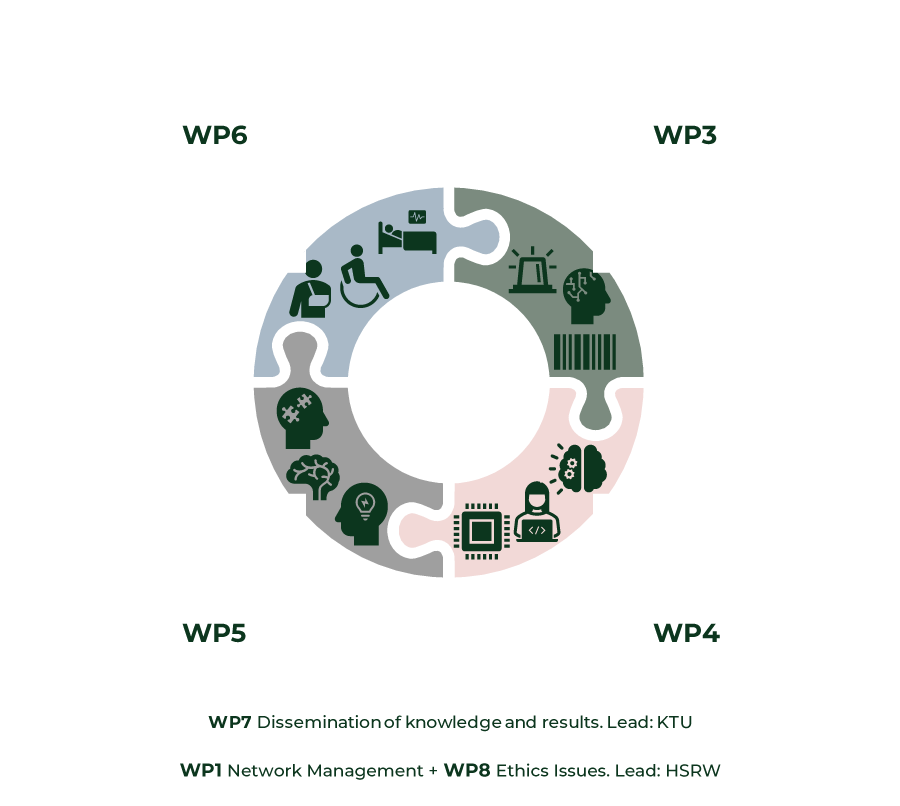
Individual Research Projects
Development of a user-friendly c-VEP-based BCI system with self-adaptation, utilizing advanced statistical and machine learning algorithms for decoding intentions during spelling. This BCI system aims for a 100% literacy rate by combining previous research with c-VEP-based BCI.
Designing a new hybrid BCI stimulus by integrating c-VEP and SSMVEP paradigms, addressing the limitations of each method. In addition, this stimulus adopts its training templates based on the live EEG data, making it more suitable for prolonged use and improving real-life user experience.
Development of an EEG-based BCI software and hardware system that can be used as a biometric authenticator. This can be utilised in various industries, nuclear facilities, airports etc. to ensure security.
Developing a user-friendly BCI visual stimulus with an optimized closed-loop parameter adjustment, that allows for individual optimized stimulation. Those parameters shall be obtained within a short experimental session, utilizing active learning strategies.
BCI systems are capable to tap into the ongoing brain state of patients while they execute a task. By repeatedly engaging users in training tasks, the BCI indicates how effectively patients are utilizing relevant brain networks for task performance. By presenting feedback to the patient the BCI system encourages better engagement of brain networks, even in damaged areas. This study investigates whether BCI users can learn to estimate the BCI output through introspection, thereby contributing to the understanding of users' self-awareness and cognitive processes in the context of BCI usage.
Identification of non-invasive EEG-based biomarkers for early detection of Alzheimer’s disease (AD) using cognitive paradigms and resting state brain activity. The hypothesis is, that certain ERP components, evoked during a modified visual oddball paradigm can be used to distinguish between SCD subjects at high or low risk of AD development.
Designing and developing new kinds of BMIs based on motor imagery for controlling lower-limb exoskeletons. The developed protocol should allow for the exoskeleton to stop or start walking without requiring any acoustic cue. Additionally, by applying deep Transfer Learning techniques the adaption of new BMI users is accelerated.
Development of a generic and low power solution for continuous impedance measurements in wearable EEG device for user-friendly everyday use. As a part of this development an integrated circuit suitable for high impedance measurements must be designed.
Exploring methods for recognition and prediction of externally expressive emotions by using hybrid neuronal (EEG and EMG) interface. A deep learning model system can be used to train on smaller datasets while maintaining generalization despite noisiness of signals. This data generalization can be investigated to obtain better results. Additionally, techniques for processing, analysis, integration and classification of EEG and EMG signals within the context of a serios game for VR environment will be developed.
Development and testing of ne BCI-HMD protocols for rehabilitation of impaired motor function after stroke to improve the understanding of brain processes associated with activities in BCI environment. This will be done by combining existing neurophysiological knowledge with the information conducted by advanced machine learning tools processing the recorded brain activities.
Work Packages (WPs)
Objectives: The Management Board of the DN network is committed to ensuring the seamless execution of DONUT through ongoing communication with the Supervisory Board. The Project Management is entrusted with critical responsibilities, including achieving project goals, ensuring rigorous quality control and effective risk management, providing administrative and financial coordination, establishing robust communication mechanisms, and securing ethical approvals. Furthermore, the management is dedicated to upholding ethical standards throughout the project and facilitating the exploitation of research outcomes.
Objectives: The focus of this work package is knowledge transfer and training activities for all partners and beneficiaries, while utilizing online training tools. A Supervisory Board comprising representatives from each beneficiary and elected DCs will monitor the quality of scientific and transferrable training activities, supervise DCs, and facilitate open communication and exchange within the project. Additionally, it focuses on network-wide training in science and complementary abilities, contributing to the overall development and growth of individual participants throughout the project.
Objectives: Within Work Package 3, our focus lies on the development of an innovative hybrid visual stimulation technique. This involves the amalgamation of various Visual Evoked Potential (VEP) types with the primary aim of minimizing visual fatigue. To achieve this goal, rigorous testing of the newly developed VEP will be conducted on a cohort of healthy participants. The objective is to ascertain whether the performance of this hybrid VEP is comparable to or even surpasses that of traditional VEPs. These trials are crucial for validating the effectiveness and efficiency of the novel visual stimulation technique, ensuring its potential application in future BCI technologies and human-machine interfaces.
Objectives: Work Package 4 centres on the design of a low-powered solution for Continuous EEG Impedance Measurement (CEIM) during recording. The initial phase involves deriving insights from this task to inform the subsequent development and testing of a portable CEIM solution. This work package is not only concerned with addressing the power consumption aspect but also concentrates on the design and testing of Brain-Computer Interface (BCI) system(s), specifically tailored for deployment as a biometric authenticator. The comprehensive approach aims to create an integrated system that not only optimizes power usage in CEIM but also advances the functionality of BCIs for secure and reliable biometric authentication purposes.
Objectives: Work Package 5 is dedicated to the development of a multifaceted Brain-Computer Interface (BCI) system with dual objectives. Firstly, the creation of a BCI system geared towards the early detection of Alzheimer's Disease is a primary focus. This involves the integration of specialized algorithms and features tailored for early indicators of the disease. Simultaneously, the work package undertakes the development of a deep learning model for expressive emotion recognition. Rigorous testing on patient datasets will be conducted to refine and optimize the model's accuracy and effectiveness. Moreover, this work package involves a collaborative initiative to design and test a hybrid EEG and EMG system. The integration of game scenarios further enhances its application for emotional state recognition. This holistic approach aims to contribute significantly to the realms of both medical diagnostics and emotional intelligence within the context of Brain-Computer Interface technology.
Objectives: This Work Package is dedicated to the development of sophisticated Brain-Computer Interface (BCI) systems with two primary objectives. Firstly, the creation of a BCI interface capable of controlling exoskeletons is a key focus. This involves the integration of intricate control mechanisms to facilitate seamless interaction between the user and the exoskeleton. Simultaneously, a complex BCI-Head-Mounted Display is being developed for motor rehabilitation purposes. The design aims to provide a comprehensive BCI experience tailored to the specific needs of individuals undergoing motor rehabilitation. Both interfaces undergo rigorous testing to ensure they meet the specific requirements of the target group, ensuring usability, effectiveness, and safety. In addition, employing modern transfer learning techniques, a machine-learning model for novel motor imagery Brain-Machine Interface (BMI) is being developed. This innovative approach allows for the adaptation of pre-existing knowledge to enhance the efficiency and performance of the model. Subsequent testing will be conducted to validate the effectiveness of the novel motor imagery BMI, contributing to advancements in motor control and rehabilitation technologies.
Objectives: Work Package 7 is dedicated to the systematic dissemination of knowledge and results generated throughout the project. The primary focus is to ensure efficient coordination among project partners for the widespread distribution of project goals, strategies, and research findings. This includes the publication of research outcomes through articles and the utilization of diverse communication channels and multimedia releases. By employing these methods, the project aims to reach a broad audience and contribute to the scientific community's collective knowledge. Furthermore, the dissemination strategy involves active collaboration with target users during the study design phase. This ensures that the outcomes of the work are not only communicated effectively but are also tailored to meet the specific needs and perspectives of the intended audience. In addition to engaging with target users, collaboration extends to other projects and networks, fostering a comprehensive exchange of insights and fostering a collective advancement in the field. This collaborative approach enhances the impact and reach of the project's outcomes beyond the immediate scope, contributing to the broader scientific and technological community.
Objectives: The last Work Package 8 focuses on ensuring ethical standards in the DONUT project. Testing BCI prototypes on human subjects follows strict ethical guidelines, aligning with EU and national legislation across seven European sites. The informed consent process includes clear criteria for participation, emphasizing understanding, voluntariness, and acknowledging no health benefits or risks. Data collection adheres to the Declaration of Helsinki, ensuring immediate pseudonymization. To maintain confidentiality, a secure token system handles participant withdrawal. Consent forms, storing critical data, are securely stored and accessible only to the experimenter. Anonymized data aligns with open science requirements. Doctoral candidates and supervisors must obtain local ethical approvals, emphasizing the highest standards. Confirmation of an appointed ethics advisor and commitment to delivering regular ethics reports underscore the consortium's dedication to ethical conduct throughout the DONUT DN project.